Reinforcement learning AI is revolutionizing how we discover new laws of physics. By using trial and error, these intelligent agents explore complex environments, maximizing rewards through their actions. They rapidly identify hidden patterns in vast datasets and formulate thousands of equations, leading to significant scientific breakthroughs. Significantly, AI has rediscovered fundamental laws, reshaping our understanding of physics. This integration of advanced algorithms turns AI from a simple tool into an essential partner in research. You'll uncover fascinating insights into this evolution and its implications for the future of scientific inquiry.
Key Takeaways
- Reinforcement learning enables AI agents to explore environments, refining their strategies for maximizing rewards through trial and error.
- AI autonomously formulates and tests hypotheses, deriving new physical laws from empirical data at remarkable speeds.
- Advanced algorithms like PhySO can generate thousands of equations per second, uncovering hidden relationships in complex datasets.
- AI has successfully rediscovered fundamental laws of physics, including Newton's second law, enhancing theoretical exploration.
Understanding Reinforcement Learning
Reinforcement learning lets agents learn from their actions in an environment, maximizing rewards through trial and error. In this framework, you can see how agents receive feedback in the form of rewards or penalties, guiding them to refine their decision-making strategies. By leveraging algorithms like Q-learning or deep Q-networks, you enable these agents to discover ideal policies for various tasks.
When applied to physical systems, reinforcement learning shines in its ability to explore complex environments and uncover new physical laws. You might think of it as a virtual laboratory where agents experiment and learn from their interactions. This iterative process leads to significant scientific discoveries, as agents can simulate scenarios that would be impractical or impossible to test in the real world.
Understanding reinforcement learning helps you appreciate its potential in advancing our grasp of the natural world. As agents navigate through environments, they not only enhance existing systems but also expand the frontiers of knowledge by identifying relationships and patterns that were previously unnoticed. This synergy between reinforcement learning and physics research opens doors to revolutionary insights into the laws that govern our universe.
AI's Role in Physics Discovery
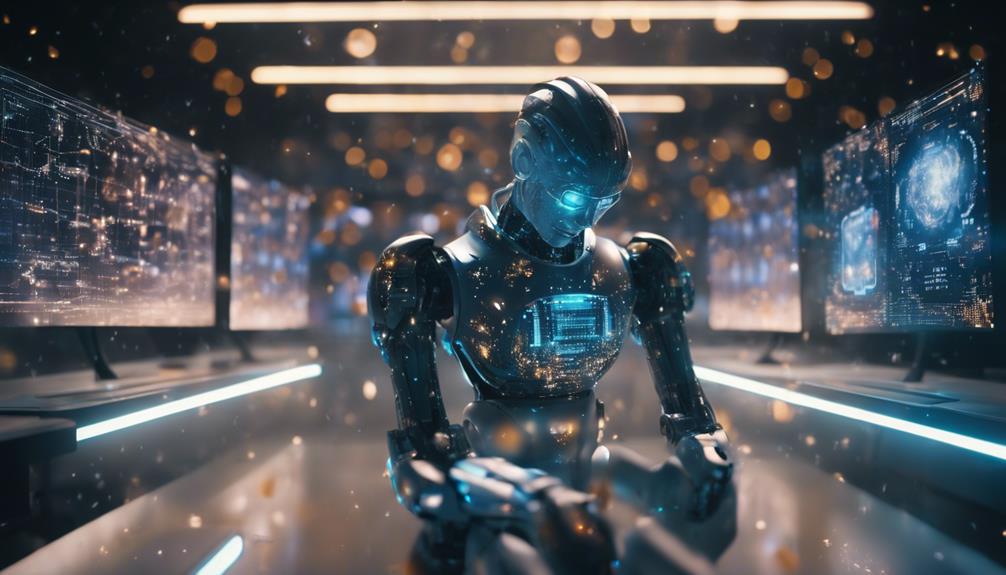
AI is reshaping the landscape of physics discovery by autonomously identifying new laws and relationships through advanced methodologies. Utilizing machine learning techniques, particularly reinforcement learning, AI can formulate and test hypotheses at an unprecedented pace. This capability allows you to explore vast datasets, like those from particle accelerators, and uncover hidden patterns that could indicate new physics.
One notable development is the PhySO (Physical Symbolic Optimization), which derives thousands of equations per second from empirical data. These innovations have even led to the rediscovery of fundamental laws, such as Newton's second law, showcasing AI's potential in theoretical physics.
AI Methodology | Impact on Physics |
---|---|
Reinforcement Learning | Formulates and tests hypotheses autonomously |
PhySO | Derives thousands of equations quickly |
Data Analysis | Uncovers patterns and anomalies |
The integration of AI into the scientific process transforms it from a mere tool to a pivotal partner in theoretical exploration, enhancing your understanding of the universe and expanding the frontiers of physics.
Historical Perspectives on AI and Physics
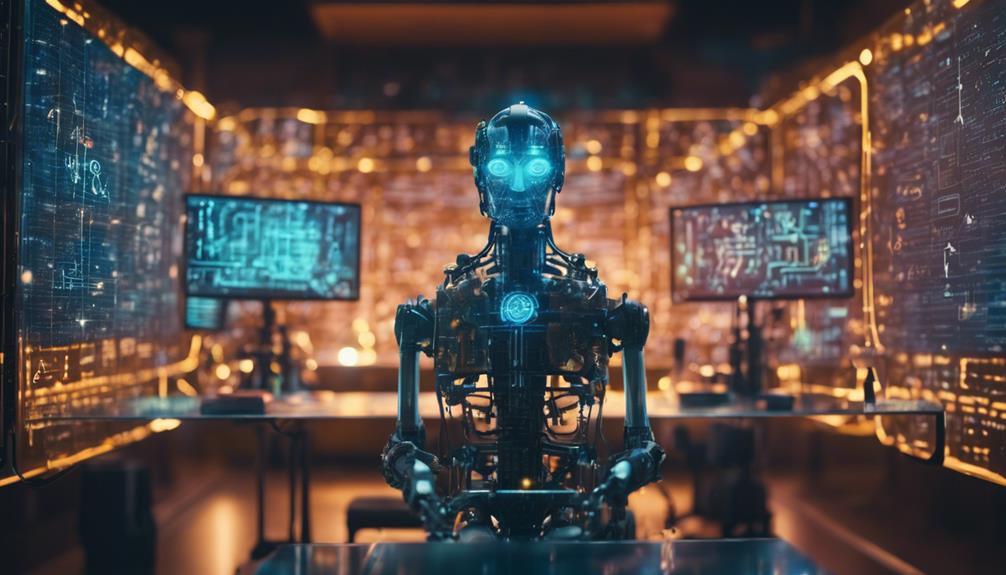
The journey of integrating artificial intelligence into physics has evolved greatly since Stephen Hawking's early predictions in 1980, highlighting a growing synergy between computational power and theoretical exploration. Hawking believed that computers would greatly aid theorists, emphasizing that AI would complement rather than replace human insight.
However, early AI applications raised concerns due to their limited explanatory power, with deep-learning algorithms producing results without elucidating underlying principles.
As AI technology advanced, its role shifted from merely analyzing data to actively discovering fundamental physical laws. For instance, recent developments have enabled AI systems to communicate in the language of physicists, uncovering hidden patterns in vast datasets. This evolution marks a pivotal moment in the scientific process, as AI's capacity to rediscover laws like Newton's second law indicates a deeper engagement with the laws of nature.
The collaboration between AI and theoretical physicists represents a potential paradigm shift, reminiscent of historical advancements in the discipline driven by computational tools. By embracing this integration, you're witnessing a transformative phase in physics, where artificial intelligence not only assists but actively participates in the quest for understanding the universe.
Advances in AI Algorithms
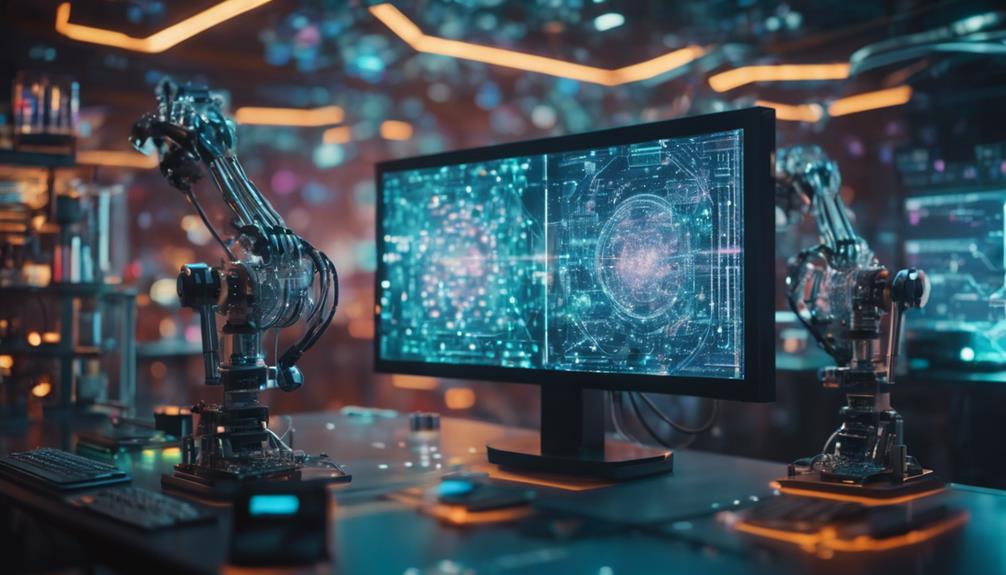
Recent innovations in algorithms have revolutionized how researchers generate and optimize physical equations from experimental data.
You'll find that reinforcement learning algorithms, like PhySO, can produce and refine thousands of physical equations each second. These algorithms employ deep reinforcement learning and dimensional analysis, streamlining the discovery process and cutting down on the combinatorial complexity typically involved in formulating physical laws.
Neural networks have further enhanced this process by effectively communicating in the language of physics. This capability allows them to identify hidden patterns within vast datasets, making it easier to uncover relationships that traditional methods might overlook. With these advances, AI systems have even successfully rediscovered established physical laws, like Newton's second law, showcasing their potential for empirical equation formulation that aligns with observable phenomena.
The integration of AI and reinforcement learning in physics research opens exciting pathways for significant breakthroughs. As you explore deeper into this domain, expect to see new laws and relationships emerge from complex physical systems, transforming our understanding of the universe.
This evolution in algorithms marks a pivotal moment for both AI and the field of physics.
Challenges Faced by AI in Physics
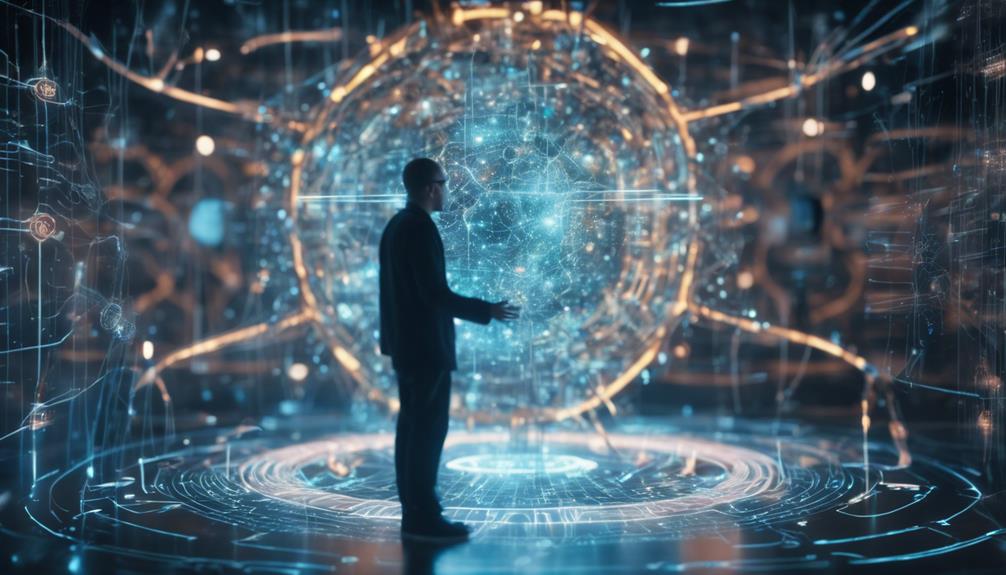
When you explore the challenges AI faces in physics, you'll notice that data quality plays a vital role in its effectiveness.
You might also find that the interpretability of AI models can hinder your understanding of how they reach conclusions.
Additionally, identifying the right variables can be a complex task, complicating the process of formulating accurate physical laws.
Data Quality Limitations
High-quality data is essential for training neural networks, yet its scarcity often limits AI's ability to explore complex and rare physical phenomena. When you think about data analysis in physics, you'll notice that the availability of experimental data varies greatly across different fields. This inconsistency can hinder AI's effectiveness in analyzing certain physical systems, especially those that lack extensive historical data.
Without a robust dataset, AI struggles to form a theoretical understanding of phenomena outside its training scope. The reliance on existing data means that innovative leaps—often sparked by human intuition—might be difficult for AI to replicate. Consequently, its generalization capabilities may remain confined to specific problems, reducing its potential to discover new laws of physics.
Collaborations are emerging to address these data quality limitations, aiming to share resources and improve the datasets available for AI training. These partnerships can enhance the overall quality of the data, leading to more accurate predictions and insights.
Ultimately, overcoming these data challenges is vital for AI to advance its role in the exploration of physics and contribute meaningfully to our understanding of the universe.
Interpretability of Models
How can AI models, often seen as 'black boxes', provide the clarity physicists need to trust their predictions and insights? The interpretability of these models is a significant hurdle, especially in understanding how they analyze complex physical systems.
Deep learning networks can produce impressive results, but their opaque nature makes it challenging to decipher the reasoning behind their outputs. You might find that AI struggles with fundamental mathematical operations, raising concerns about the reliability of its predictions in physics.
When it comes to maintaining coherence in the models it generates, the lack of interpretability can make the process even more frustrating. Dimensional analysis helps manage the combinatorial complexity of possible equations, yet it often restricts the mathematical combinations the AI can explore.
Without a clear understanding of how an AI arrives at its conclusions, physicists may hesitate to embrace its findings. The quest for ideal equations can quickly devolve into chaos without accessible insights.
Therefore, ensuring that AI-generated models are interpretable is essential for fostering trust and advancing research in the domain of physical systems.
Variable Identification Challenges
AI systems struggle to accurately identify and correlate variables in complex physical systems, complicating their ability to provide reliable insights. You might find it surprising that AI initially identified an average of just 4.7 variables for double pendula, making it difficult to relate these to known quantities. This highlights the variable identification challenges faced by AI when dealing with complex phenomena.
Moreover, the non-intuitive nature of AI-generated variable descriptions can lead to confusion, especially when valid predictions arise for different systems. During experiments with chaotic systems, the number of state variables varied considerably—8 for inflatable tube men and 24 for a fireplace—indicating that multiple valid descriptions may exist for the same phenomena. This inconsistency suggests that our understanding of fundamental variables in physics might still be incomplete.
AI's struggle with basic mathematical operations, like addition and division, adds another layer of complexity. This emphasizes the need for improved interpretability in the neural networks used for physics research, as clearer models can enhance AI's effectiveness in exploring theoretical concepts within complex physical systems.
Rediscovery of Fundamental Laws
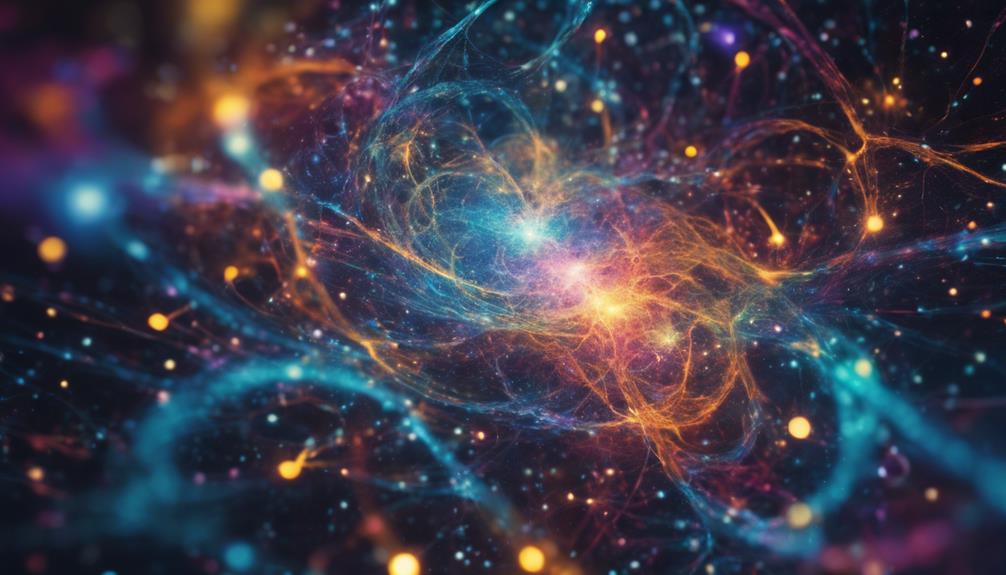
Researchers are excited by the AI's ability to rediscover 74 fundamental physical laws, revealing new insights into established scientific principles. By leveraging deep reinforcement learning, the AI analyzed extensive datasets to identify patterns that human researchers might overlook. This method allowed it to formulate thousands of equations per second while ensuring dimensional analysis streamlined the process.
Among the rediscovered laws is the harmonic oscillator, a cornerstone of physics that describes various physical systems. The AI's capability to align empirical equations with observational needs, as highlighted in a study published in 'The Astrophysical Journal' on December 11, 2023, has garnered significant attention, especially on social media.
The importance of this rediscovery can't be overstated—AI isn't just generating new equations; it's enhancing our understanding of existing physics. This innovative approach serves to complement traditional methods, providing a fresh lens to examine and validate established scientific principles.
With the AI's success, the physics community is now more optimistic about the future of research, as it opens doors to deeper investigations of physical laws that govern our universe.
Future Implications of AI Integration
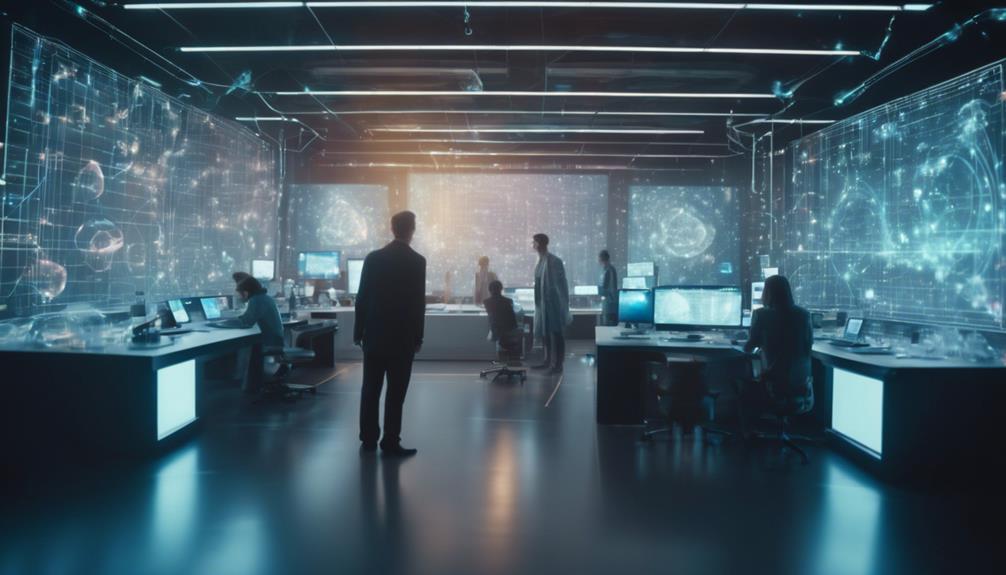
The recent successes in rediscovering fundamental laws of physics highlight the promising future implications of integrating AI into research, paving the way for groundbreaking discoveries and enhanced collaboration in the field.
By employing reinforcement learning methodologies like PhySO, you can expect AI to generate new insights at an unprecedented pace, formulating thousands of equations per second. This capability allows AI to analyze extensive datasets, pushing the boundaries of what's currently possible in theoretical physics.
As AI evolves, its potential to communicate findings in a way that aligns with physicist methodologies will likely foster collaboration among researchers, breaking down traditional silos. You might find AI exploring uncharted territories, such as dark matter and gravitational waves, leading to paradigm shifts in our understanding of the universe.
Moreover, integrating AI into physics research opens doors to innovative approaches that can reshape how you approach scientific questions. The ability to uncover new laws governing physical phenomena could revolutionize the field, making previously unimaginable discoveries a reality.
Embracing AI's potential won't only enhance your research capabilities but also redefine the landscape of theoretical physics for generations to come.
Ethical Considerations in AI Research
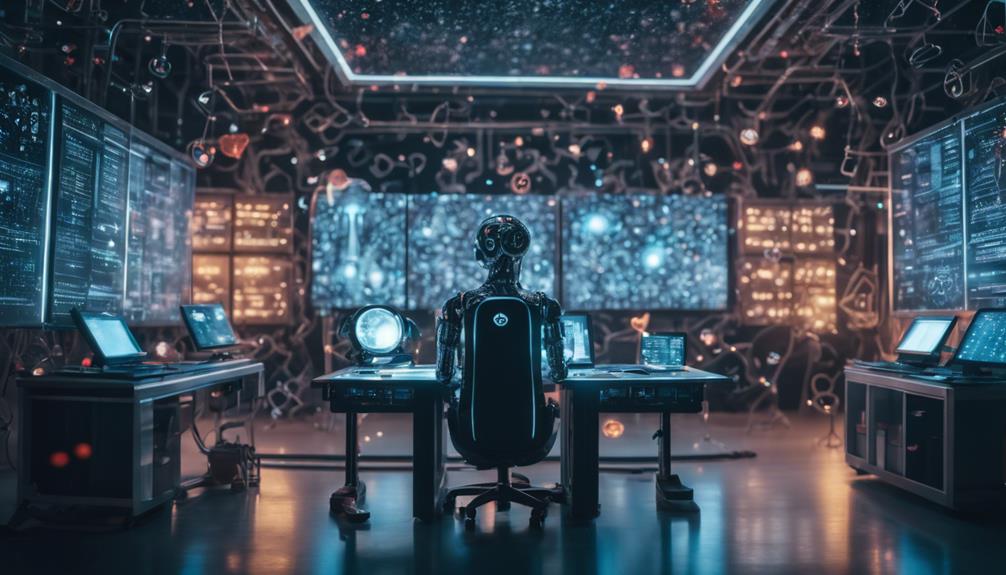
Understanding the ethical considerations in AI research is fundamental, especially when it comes to uncovering new laws of physics that can reshape our scientific landscape. You need to be aware that transparency in AI decision-making processes is critical. When AI generates findings, the implications can greatly impact scientific understanding, so it's imperative to guarantee that these processes are clear and accountable.
Moreover, consider the potential biases in training datasets used for reinforcement learning. If AI inadvertently perpetuates these biases, it could lead to unethical scientific conclusions. This highlights the importance of ongoing dialogue about human oversight in AI-driven research. Relying solely on AI might undermine the integrity of your findings.
As you explore AI's ability to generate new scientific knowledge, remember the responsibility to accurately attribute discoveries. It's your duty to guarantee that this technology serves the greater good of society.
Frequently Asked Questions
Can AI Discover New Laws of Physics?
Yes, AI can discover new laws of physics. By analyzing vast amounts of data and identifying patterns, it explores relationships that humans might miss, potentially leading to groundbreaking insights and a deeper understanding of the universe.
Does AI Learn Through Trial and Error?
Yes, AI learns through trial and error. You'll see it explore different actions, you'll watch it adjust strategies, and you'll notice it improve decision-making as it receives feedback to maximize rewards over time.
What Is the AI Technique That Used Rewards and Penalties to Learn Through Trial and Error?
The AI technique you're referring to is reinforcement learning. It uses a system of rewards and penalties to guide agents, helping them learn ideal behaviors through trial and error in various environments.
What Is the Learning Methodology Where the Model Learns Through a Trial and Error Method?
You're referring to the trial and error learning methodology, where you explore different actions, receive feedback, and adjust your approach based on successes and failures, ultimately refining your understanding and improving decision-making over time.
How Did the Reinforcement Learning AI Discover the New Laws of Physics?
The reinforcement learning ai creates new matter by discovering the new laws of physics through continuous experimentation and analysis. By processing vast amounts of data and running simulations, the AI is able to find patterns and connections that elude human scientists, opening up new frontiers in our understanding of the universe.
Conclusion
In a world where curiosity knows no bounds, your exploration of AI in physics reveals a thrilling frontier. As reinforcement learning uncovers new laws through trial and error, you stand on the brink of scientific evolution.
This fusion of technology and discovery not only reshapes your understanding of the universe but also sparks a conversation about the ethics that accompany such power.
Embrace this journey, for the future of physics is a canvas waiting for your brushstrokes.