Reinforcement learning algorithms are transforming city traffic management, and you can expect markedly reduced commuting times. By optimizing traffic signals in real-time, these systems minimize congestion and enhance flow. This means you'll experience fewer stops and smoother journeys. In fact, some studies show a potential for cutting daily commutes in half by improving traffic efficiency. Not only does this tech streamline daily travel, but it also boosts emergency response times and lowers carbon emissions. If you want to explore how these innovations might affect your city, there's so much more to uncover.
Key Takeaways
- Reinforcement learning optimizes traffic signals in real-time, significantly reducing congestion and improving overall urban mobility.
- Emergency vehicle prioritization through RL leads to faster response times, cutting average emergency stop durations by 44.16%.
- Dynamic adjustments of traffic light cycles based on real-time data can halve commute times during peak traffic conditions.
- Implementing RL in cities has proven to enhance travel efficiency and reduce waiting times, contributing to smarter urban environments.
Overview of Reinforcement Learning
What if you could teach a computer to make better decisions by rewarding it for the right choices? That's the essence of Reinforcement Learning (RL). In RL, an agent learns to navigate its environment by receiving rewards or penalties based on its actions.
This concept is particularly powerful when applied to traffic signal control systems. By utilizing traffic flow data, RL algorithms can optimize traffic signals in real-time, adapting to current conditions to minimize congestion levels at intersections.
Imagine automated systems that adjust traffic lights based on live data from vehicles and pedestrians. These intelligent transportation systems can greatly enhance urban mobility by reducing waiting times and improving overall traffic flow.
Studies have shown that RL can decrease emergency stops by up to 44.16%, showcasing its effectiveness in managing complex traffic situations.
Impact on Traffic Efficiency
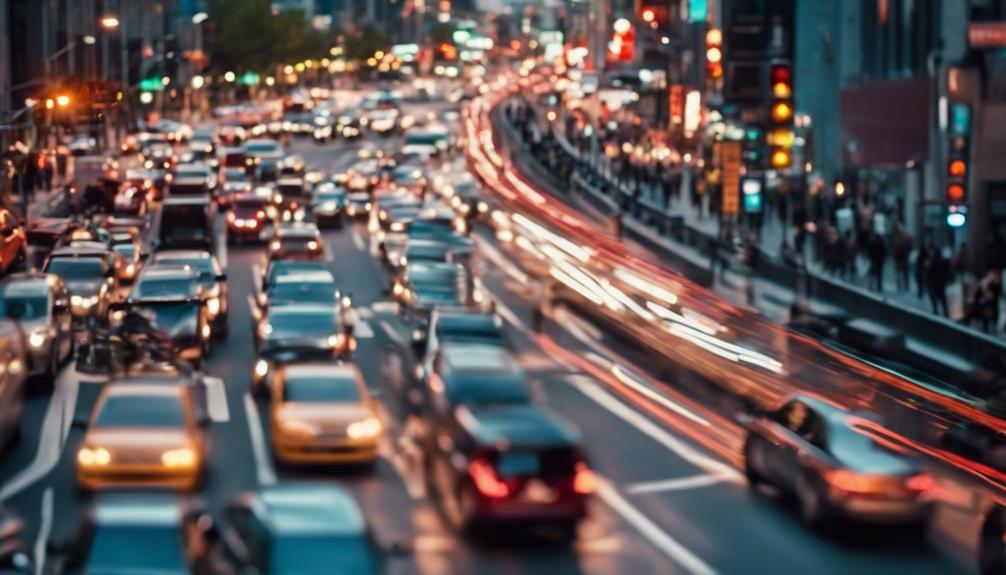
You'll notice a significant impact on traffic efficiency with the implementation of the reinforcement learning algorithm.
By reducing congestion levels and enhancing emergency response times, your daily travel will be smoother and faster.
Improved travel times not only make your commutes easier but also contribute to a more efficient urban environment.
Reduced Congestion Levels
The deployment of reinforcement learning algorithms has considerably reduced congestion levels, leading to a more efficient traffic flow in urban areas. By optimizing traffic light management through real-time adjustments, cities are now better equipped to combat traffic congestion. These machine learning models analyze current traffic conditions, allowing for the timely alteration of light cycles, which considerably improves traffic flow.
Here are some key benefits of reduced congestion levels:
- Decreased average emergency stops by 44.16%, enhancing overall traffic efficiency.
- Minimized average waiting times at traffic signals, contributing to smoother vehicular movement.
As a result, commuters experience shorter travel times and reduced frustration, ultimately enhancing the quality of life in urban environments.
With systematic improvements in traffic management, you're likely to enjoy increased free time, making your daily commute less of a chore. The ripple effect of these advancements extends beyond just traffic; they contribute to a healthier, more connected community where people can thrive.
Embracing these innovative solutions paves the way for smarter, more sustainable cities, benefiting everyone involved.
Enhanced Emergency Response
Reinforcement learning algorithms have stepped up emergency response times by optimizing traffic light cycles, allowing emergency vehicles to navigate through urban areas more efficiently. By implementing real-time adjustments, these algorithms greatly decrease average emergency stops by 44.16%, expediting response times when every second counts.
Machine learning models predict traffic congestion, enabling proactive signal changes that clear the way for emergency vehicles. This integration improves not only the effectiveness of emergency operations but also overall traffic flow, as optimized light cycles reduce idling time and congestion for all road users.
When emergency services can move quickly through a city, it enhances urban mobility by ensuring that critical response times are met without unnecessary delays. As these systems learn and adapt, they maintain a dynamic traffic management approach that keeps pace with changing conditions.
The result is a more efficient routing process for emergency responders, making it easier for them to reach their destinations swiftly. In this way, reinforcement learning not only enhances emergency response but also contributes to a more streamlined and effective urban traffic system overall.
Improved Travel Times
How can cities achieve improved travel times and enhanced traffic efficiency through advanced traffic management systems? The answer lies in leveraging machine learning algorithms and adaptive strategies to optimize traffic flow. By applying real-time traffic data, cities can dynamically adjust traffic signals, reducing congestion and making commutes smoother for everyone.
Here are a few key benefits you can expect from these improvements:
- Reduced Congestion: AI-driven systems can adapt to changing traffic patterns, considerably decreasing bottlenecks.
- Enhanced Travel Times: Studies show that automated adjustments to traffic signals can reduce average waiting times by 8%, making your daily commute faster.
The implementation of reinforcement learning algorithms not only maximizes traffic flow but also enhances urban transportation systems. This innovative approach leads to shorter travel times, making cities more efficient and enjoyable for their residents.
As cities embrace these technologies, you'll likely notice a considerable change in your daily commute, positively impacting your overall quality of life.
Data Collection and Analysis
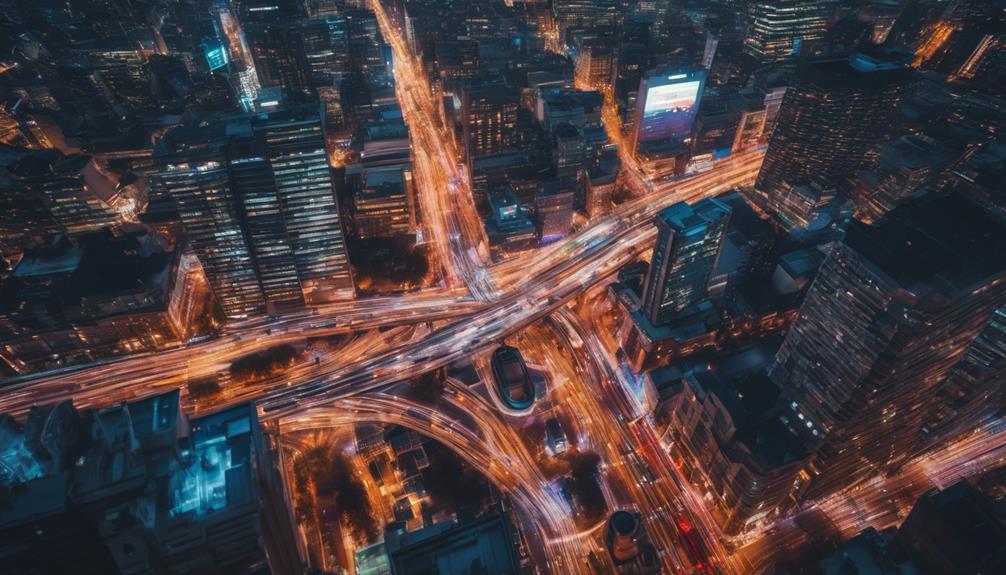
Collecting real-time traffic data from NYC DOT video cameras every two seconds allows for precise optimization of traffic light cycles along East Houston Street. These cameras capture images at 13 strategic locations, providing invaluable traffic data that reflects current conditions. By using YOLOv3, a robust dataset is created, which removes 404 outliers to guarantee accurate analysis of traffic volume and density patterns.
As you observe, traffic volume trends are categorized by time of day, revealing peak congestion during morning rush hours and a gradual decrease throughout the day. This understanding of traffic patterns informs the predictive model, which employs Machine Learning algorithms like Random Forest to analyze historical data and forecast future traffic volumes.
Additionally, the analysis incorporates local weather conditions and social media event data to enrich your understanding of real-time traffic dynamics in the urban environment. This extensive approach enhances traffic control strategies, enabling more efficient management of city traffic and ultimately improving overall commuter experiences.
Traffic Light Optimization Techniques
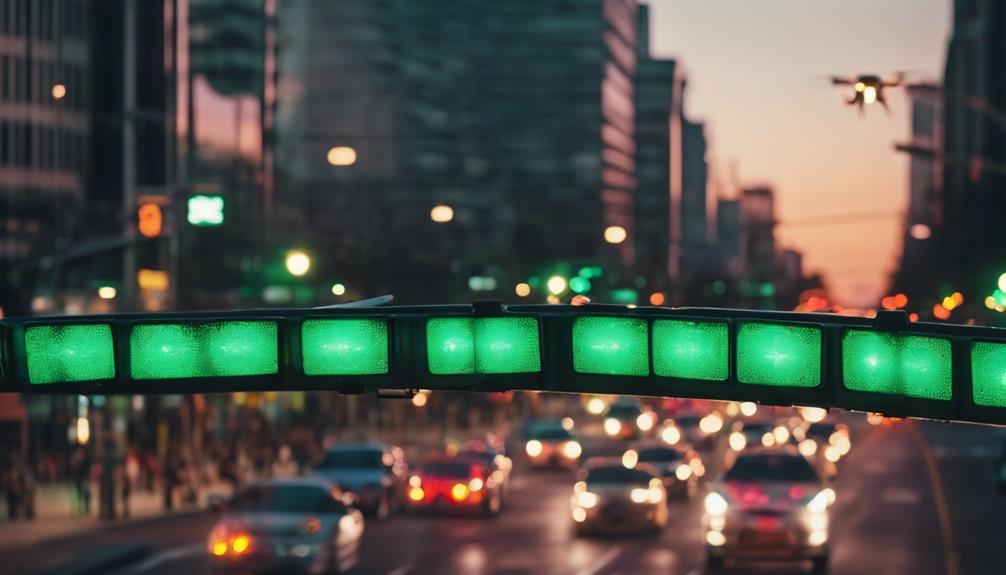
When it comes to optimizing traffic lights, you'll find that real-time signal adjustments play an essential role in improving traffic flow.
By prioritizing emergency vehicles, these techniques guarantee that first responders can reach their destinations quickly and safely.
This not only reduces congestion but also enhances overall urban mobility.
Real-Time Signal Adjustments
Reinforcement Learning algorithms are transforming traffic management by enabling real-time signal adjustments that optimize traffic light cycles for smoother flows.
With the ability to analyze historical data and adapt to current conditions, these systems actively reduce congestion and enhance the commuting experience. By employing machine learning techniques, RL can predict traffic patterns and adjust signals accordingly, greatly cutting down on waiting times.
You'll notice a marked decrease in average waiting times at traffic signals.
Real-time adjustments lead to smoother traffic flows, enhancing overall urban transportation.
Automated systems can reduce emergency stops, benefiting both drivers and emergency vehicles.
Incorporating Reinforcement Learning into traffic management systems allows cities to adapt dynamically. This results in a notable reduction in congestion, which ultimately makes urban transportation more efficient.
The integration of advanced algorithms can achieve impressive accuracy, with studies showing up to 95.7% precision in traffic light detection and control.
Emergency Vehicle Prioritization
Integrating emergency vehicle prioritization with traffic light optimization techniques enhances response times and guarantees that first responders can navigate through urban environments more efficiently.
By utilizing reinforcement learning, particularly algorithms like the Deep Q-Network (DQN), cities can greatly reduce the frequency of emergency stops by optimizing traffic signal systems. Studies have shown a 44.16% decrease in these stops, allowing emergency vehicles to move swiftly through congested areas.
The combination of real-time data and reinforcement learning enables traffic signal systems to adjust dynamically, providing quicker passage during critical situations. Advanced traffic management systems can even override standard light cycles, achieving 100% accuracy in identifying emergency vehicles through integrated object detection methods. This optimization not only aids emergency responders but also enhances overall traffic flow, benefiting all road users.
Moreover, by analyzing historical traffic data, cities can proactively adjust traffic signals, mitigating congestion and improving emergency vehicle routing. This proactive approach leads to more efficient urban mobility, ensuring that emergency services reach their destinations promptly and effectively.
Ultimately, prioritizing emergency vehicles in traffic management systems is vital for fostering safer and more responsive urban environments.
Environmental Benefits of Optimization
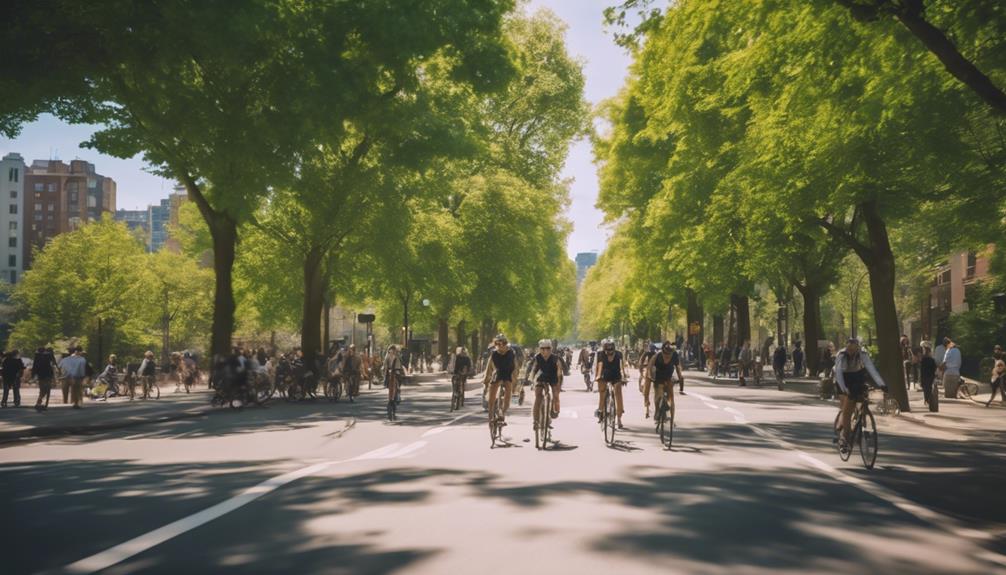
Optimized traffic light cycles can greatly cut down on vehicle idling, leading to a notable reduction in carbon emissions and improved air quality in urban areas. When you implement smart city solutions like optimizing traffic signals, you're actively combating traffic congestion and enhancing traffic flow. This results in less fuel consumption and a smaller carbon footprint.
By utilizing machine learning models for traffic management, cities can achieve significant emissions reduction. Consider these environmental benefits:
- Reduced emissions: Lower idling times lead to decreased greenhouse gas emissions from on-road vehicles.
- Improved air quality: Enhanced traffic systems contribute to cleaner air, benefiting public health.
A study revealed that 29% of NYC's emissions come from on-road vehicles, underscoring the importance of optimizing traffic signals. By enhancing traffic flow and minimizing the environmental impact of prolonged vehicle operation, we can create a more sustainable future.
Embracing these innovations not only improves daily commutes but actively contributes to a healthier planet for all.
Case Studies and Real-World Applications
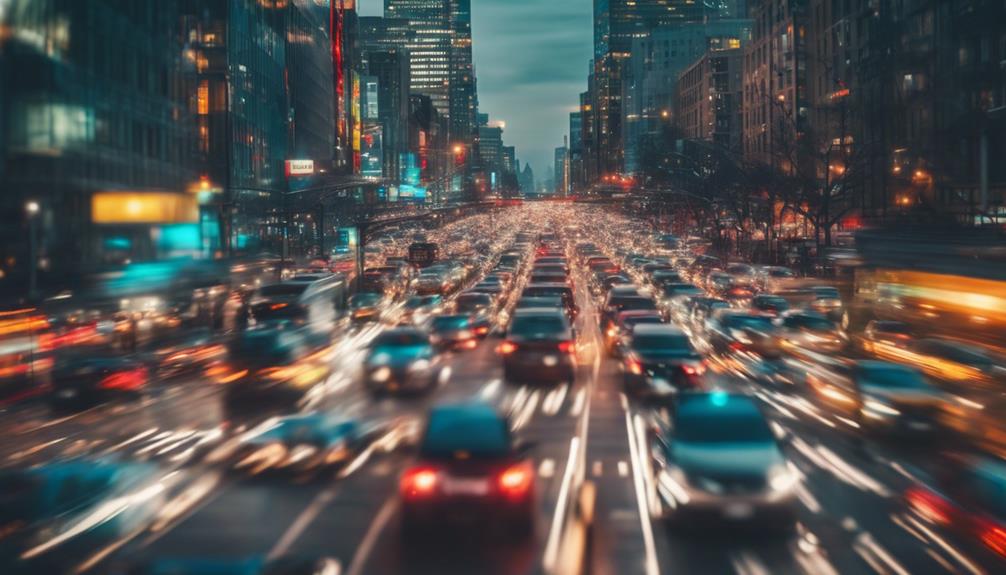
Cities around the world are implementing innovative traffic management solutions that harness advanced algorithms to tackle congestion and improve commuter experiences.
In Los Angeles, the Automated Traffic Surveillance and Control (ATSAC) system uses machine learning for the optimization of traffic signal timings, which has notably decreased travel times for commuters. Meanwhile, Singapore's Electronic Road Pricing (ERP) employs Artificial Intelligence to adjust toll rates based on real-time traffic conditions, leading to remarkable reductions in congestion.
Copenhagen's Smart Parking project showcases how AI-driven solutions can provide real-time parking availability, reducing search time and alleviating urban traffic congestion. A compelling case study utilizing a Deep Q-Network (DQN) algorithm revealed a 44.16% decrease in average emergency stops, illustrating the effectiveness of reinforcement learning in optimizing traffic light cycles. Additionally, simulation-based training with reinforcement learning approaches has shown an 8% improvement in waiting times within traffic light control systems.
These real-world applications demonstrate the transformative potential of advanced technologies in urban traffic systems, paving the way for smarter, more efficient cities.
Challenges and Ethical Considerations
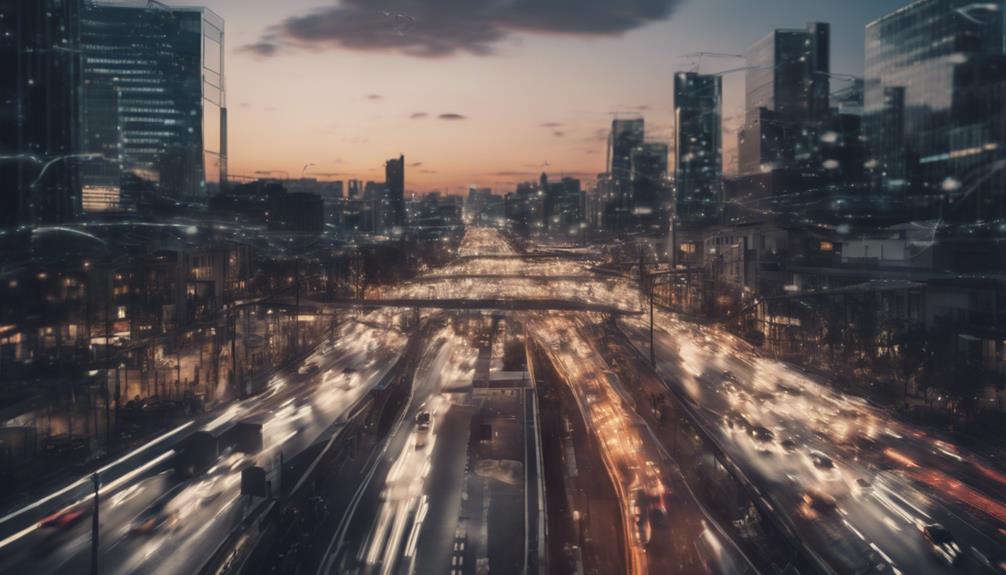
While the benefits of AI-driven traffic management are evident, significant challenges and ethical considerations must be addressed to guarantee these technologies serve all members of the community fairly.
Privacy concerns arise from extensive data collection, requiring robust cybersecurity measures to protect user information. Without proper safeguards, data breaches could erode public trust.
Algorithmic bias is another significant issue. If not properly managed, AI systems may discriminate against marginalized groups, limiting their access to improved traffic solutions. Ensuring equitable access is imperative to prevent further disparities in transportation.
Here are some key points to reflect on:
- Workforce development: The shift to AI might displace jobs in traditional transportation sectors, highlighting the need for reskilling programs.
- Accountability: Clear guidelines for data collection and sharing must prioritize privacy rights while fostering transparency in AI systems.
Addressing these challenges head-on is fundamental to ensuring that AI-driven traffic management benefits everyone and promotes a fairer, more efficient transportation system.
Future of Traffic Management
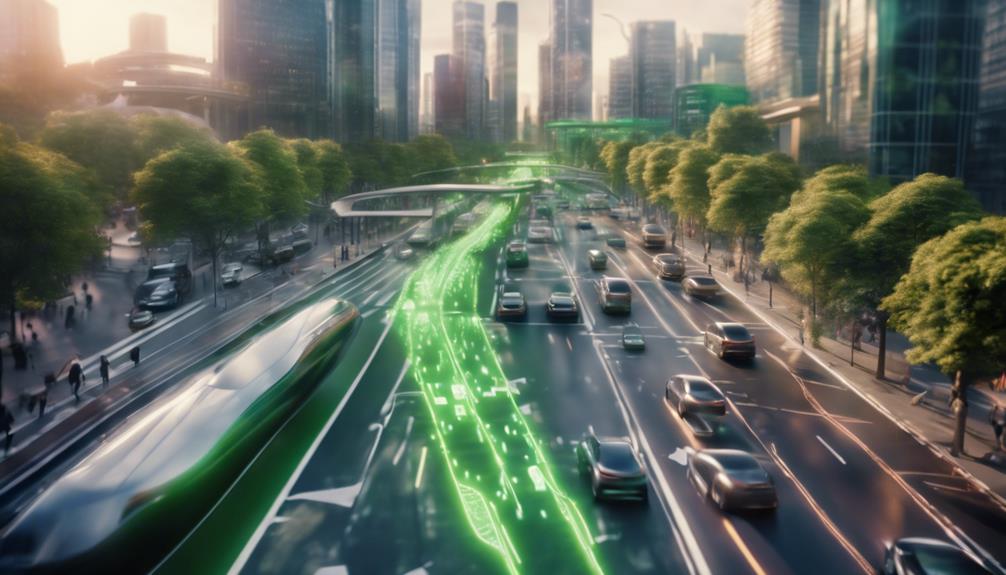
The future of traffic management promises to revolutionize urban mobility through the integration of advanced reinforcement learning algorithms and smart technology. These AI-driven systems utilize real-time data to dynamically adjust traffic signals, effectively reducing congestion and enhancing overall transportation flow.
Imagine a city where traffic lights respond instantly to changing conditions, leading to a significant decrease in average emergency stops—up to 44.16% in simulations. With the ability to adapt continuously, these smart systems optimize traffic management, cutting commute times in half and improving efficiency.
This transformation not only makes daily travel smoother but also minimizes fuel consumption and lowers emissions from idling vehicles. As we move forward, the integration of IoT devices and smart city infrastructure will further amplify the effectiveness of reinforcement learning in managing urban traffic patterns.
You'll see more streamlined traffic flow, improved resource utilization, and a cleaner environment. The combination of AI-driven technology and real-time data will shape a future where urban mobility isn't just efficient but also sustainable, paving the way for smarter, more connected cities.
Frequently Asked Questions
How to Reduce Traffic Congestion Using Ai?
To reduce traffic congestion using AI, leverage real-time data for dynamic signal adjustments, implement predictive algorithms to anticipate jams, and utilize intelligent transportation systems for efficient routing and incident detection. You'll enhance overall traffic flow considerably.
What Is the Algorithm for Traffic Prediction?
To predict traffic, you can use algorithms like regression models or reinforcement learning. They analyze historical data and real-time conditions, adjusting predictions to improve traffic management and minimize congestion effectively.
What Is the AI Model for Traffic Control?
You're exploring AI models for traffic control, and you'll find that Deep Q-Networks (DQN) optimize traffic light cycles, enhance flow, and adapt in real-time, ultimately reducing congestion and improving overall efficiency in urban settings.
How Can AI Be Used in Traffic?
You can use AI in traffic management by analyzing data from various sources to optimize signal timings, reduce congestion, and enhance emergency vehicle routing, ultimately improving efficiency, lowering emissions, and creating a more sustainable urban environment.
Conclusion
As you navigate the bustling streets, the intelligent dance of traffic lights symbolizes a new era in urban commuting.
With reinforcement learning guiding each signal, your journey transforms from frustration to fluidity, cutting your travel time in half.
This algorithm doesn't just optimize traffic; it breathes life into the city, reducing emissions and creating a vibrant environment.
Embrace this change, for each green light represents progress, illuminating a path toward a smarter, more sustainable future for all.