You've probably heard about predictive AI, like the Life2vec model, which can forecast life expectancy with an uncanny 78% accuracy. It analyzes vast datasets, including individual habits and health information, to make these predictions. While this technology can feel eerie, it shows how data-driven insights can inform personal decisions. However, it's vital to understand the limitations and ethical concerns surrounding its use. This blend of prediction and potential pitfalls makes the conversation about AI both fascinating and significant. If you're curious about its implications and future, there's much more to uncover.
Key Takeaways
- The Life2vec model predicts life expectancy with 78% accuracy by analyzing data from over 6 million individuals, including various life factors.
- It utilizes extensive datasets, including health and economic data, to enhance the accuracy of mortality forecasts within four years.
- The model's predictions are based on historical data, but individual circumstances create a 22% margin of error in forecasts.
- Public perception of AI predictions often involves anxiety due to misunderstandings about AI's capabilities and the nature of its predictions.
Overview of Predictive AI
Predictive AI harnesses vast datasets to forecast outcomes like life expectancy and financial security, transforming how you comprehend individual and societal trends. By utilizing extensive datasets from over 6 million individuals, models such as Life2vec can predict life expectancy with an impressive accuracy of 78%. This technology analyzes numerous factors, including income, profession, smoking habits, and exercise routines, to uncover patterns that notably influence mortality predictions.
At the heart of this innovation are transformer models, which are designed to process large-scale life event data efficiently. These models, mainly used in natural language processing, enable Predictive AI to sift through complex data sets and identify correlations that might go unnoticed through traditional analysis. The research, primarily conducted in Denmark from 2008 to 2016, provides a solid foundation for these predictions, focusing on life events and health data during that period.
However, while the potential benefits are immense, ethical considerations arise regarding privacy and the psychological impact on individuals aware of their predicted outcomes. As you navigate this evolving landscape, it's essential to weigh these implications alongside the transformative possibilities Predictive AI offers.
Methodology Behind Predictions
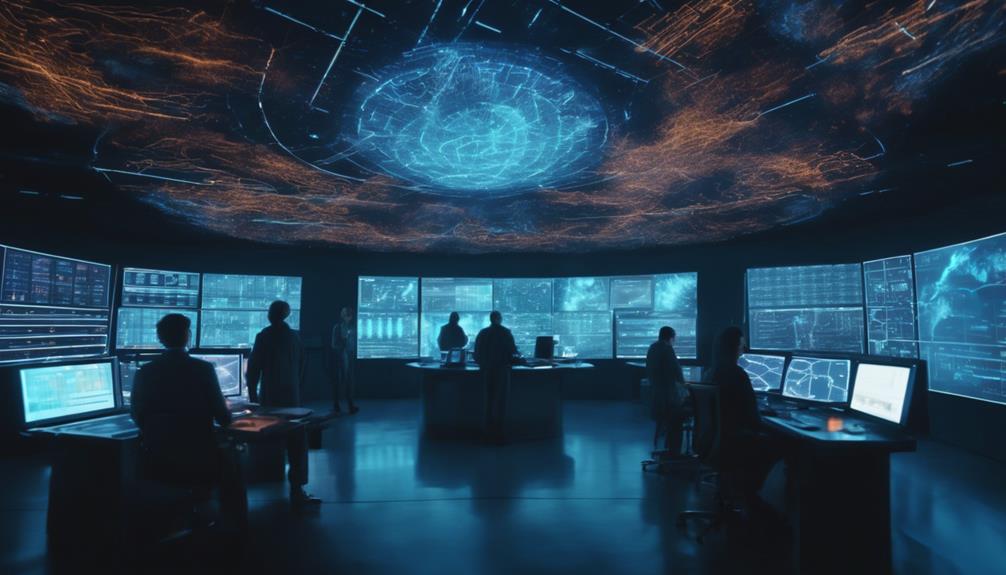
In exploring the methodology behind predictions, you'll see how data sources play a vital role in shaping outcomes.
The Life2vec model uses extensive datasets from millions of individuals to boost its prediction accuracy.
Data Sources Utilized
The AI tool Life2vec taps into rich datasets from over 6 million Danish individuals, focusing on life events and health records to enhance its mortality predictions. It utilizes extensive health data from the National Patient Registry, capturing various factors such as income, job type, healthcare visits, and medical diagnoses. By encoding life events into a vector space, the algorithm identifies patterns that influence life expectancy and financial status at death.
Here's a breakdown of the data sources used in Life2vec:
Data Source | Description |
---|---|
National Patient Registry | Contains health data, including medical diagnoses and visits. |
Economic Data | Includes income and job type information relevant to mortality. |
Life Events | Records significant life events from 2008 to 2016. |
These diverse data points allow Life2vec to train transformer models, similar to those used in natural language processing, ensuring a robust framework for mortality prediction. This methodology achieves an impressive accuracy rate of 78% for forecasting mortality within four years, showcasing the power of integrated data in predictive analytics.
Prediction Accuracy Metrics
To gauge mortality forecasts accurately, Life2vec employs a sophisticated methodology that combines various data points, ensuring you're getting insights based on thorough analyses.
The model, which draws from data on over 6 million Danish individuals, boasts an impressive prediction accuracy rate of 78% for mortality forecasts within a four-year timeframe.
Life2vec's algorithm scrutinizes multiple factors, such as income, profession, smoking habits, exercise routines, and medical history, to identify patterns that influence life expectancy. By concentrating on life-events gathered between 2008 and 2016, it focuses on a demographic aged 35 to 65, many of whom have passed away by the study's conclusion.
These life events are encoded in a vector space, allowing structured analysis via transformer models that learn from extensive datasets to refine predictive capabilities.
However, it's crucial to recognize the methodology's limitations. While predictions can achieve high accuracy, individual circumstances and the unpredictable nature of life events can greatly impact outcomes.
Therefore, while Life2vec offers valuable insights into human mortality, you're reminded that predictions aren't absolute certainties.
Public Perception of AI Predictions
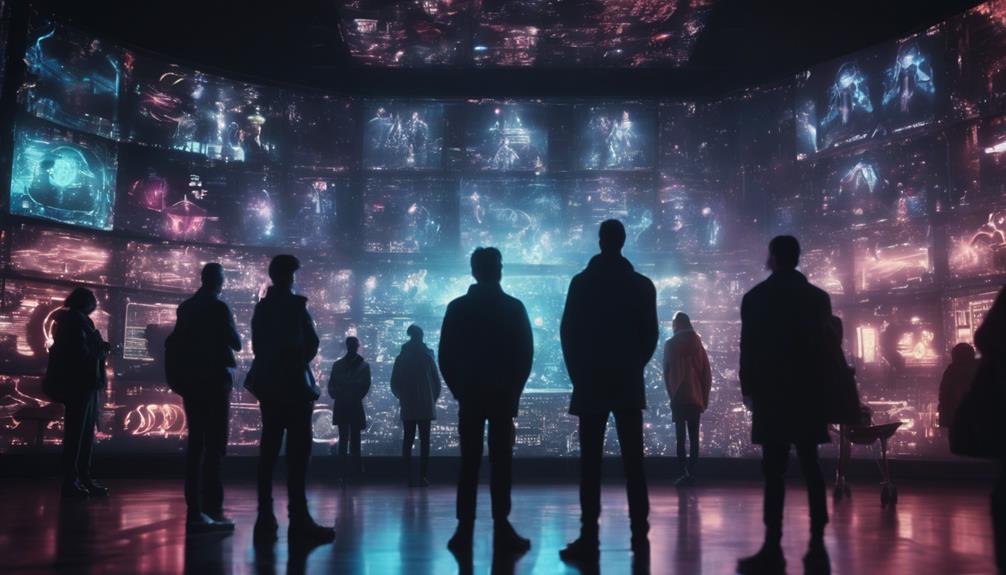
When you think about AI predictions, it's easy to feel both intrigued and anxious. Many people worry about unknown outcomes, leading to misconceptions about what AI can really do.
As you grapple with these feelings, it's important to reflect on how media and public discourse shape your understanding of AI's true capabilities.
Fear of Unknown Outcomes
Public fascination with AI's predictive abilities often collides with deep-seated fears about the unknown, especially regarding life-altering events like death. You might find yourself intrigued by how accurately AI can predict certain outcomes, yet uneasy about what those predictions mean for your life. The idea that a model like Life2vec can predict mortality with 78% accuracy can evoke a strong fear, especially when faced with the thought of knowing your own death date.
This fear is compounded by the psychological impacts of such predictions. When you're confronted with potential outcomes regarding your health or financial status, anxiety can spike. You may begin to question your choices and behaviors, altering your life based on forecasts that mightn't fully capture your reality.
It's essential to understand that while AI can predict trends based on data, it doesn't account for the complexities of individual lives. The media often amplifies these fears, creating a narrative that AI can foresee the future with unfaltering precision. Educating yourself about the limitations of AI tools can help you navigate these fears and make informed decisions about how you engage with predictive technologies.
Misconceptions About AI Abilities
Many people mistakenly believe that AI can predict individual events with certainty, overlooking its reliance on historical data and statistical patterns. This misunderstanding fosters several misconceptions about AI abilities, especially regarding its predictive power in life and death scenarios. For instance, when discussing AI models like the life and death calculator, it's essential to recognize that these tools aren't infallible.
Here are three key misconceptions you should be aware of:
- AI Can Guarantee Outcomes: Many think AI predictions are certainties, but they're based on probabilities and trends, not exact predictions.
- Media Exaggeration: Sensationalized stories often portray AI as possessing supernatural foresight, which can distort your understanding of its actual capabilities.
- Fear of AI's Predictions: Misconceptions about AI's abilities can lead to unnecessary anxiety about predicted outcomes, overshadowing the fact that these models are merely tools for analysis.
Ethical Considerations of AI
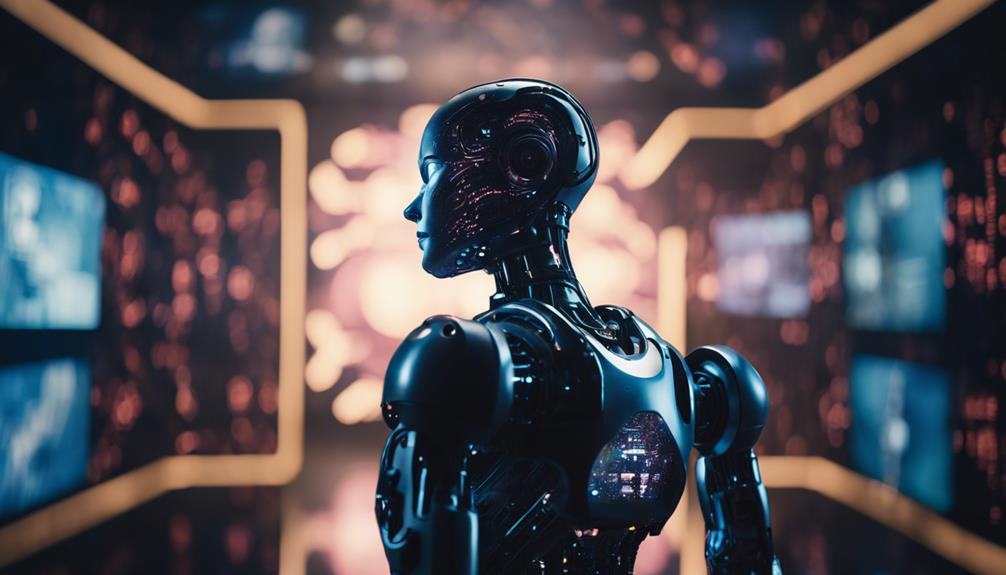
As AI tools like Life2vec gain traction, ethical considerations surrounding data privacy, user consent, and potential discrimination become increasingly critical. The reliance on extensive datasets from over 6 million individuals raises significant concerns about how your data is used and shared. You might feel anxious or fearful knowing that your life expectancy or financial status could be predicted, affecting your mental health and overall well-being.
To illustrate these ethical concerns, consider the following table:
Ethical Concern | Description | Impact |
---|---|---|
Data Privacy | Protection of personal information against misuse | Risk of identity theft |
User Consent | Guaranteeing individuals agree to data usage | Transparency in data handling |
Discrimination | Potential bias in predictions affecting judgment | Unfair treatment in society |
Regulatory Frameworks | Need for laws governing AI use in sensitive areas | Protection against exploitation |
Danish laws already prohibit the use of predictive data in insurance and hiring, showcasing the urgent need for regulatory frameworks to safeguard individuals. Ongoing discussions stress the importance of developing ethical guidelines that promote responsible AI deployment.
Accuracy and Reliability of Predictions
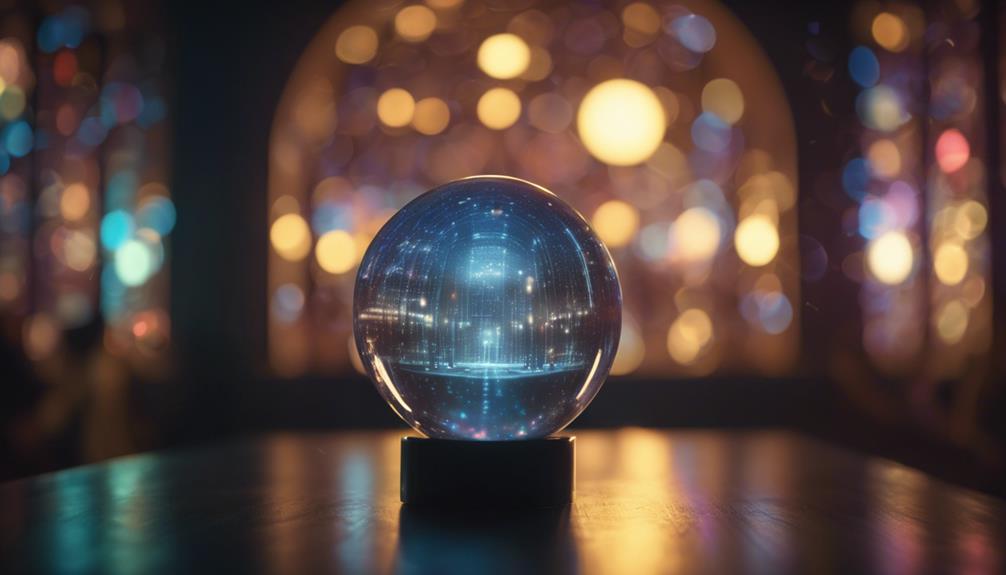
While ethical deliberations surrounding AI raise important questions, understanding the accuracy and reliability of predictions made by models like Life2vec is equally essential for users who rely on these insights.
Life2vec has achieved an impressive prediction accuracy rate of 78% in forecasting mortality over a four-year period, based on extensive data from 6 million Danish individuals. This level of accuracy stems from analyzing various influencing factors, such as income, profession, smoking habits, exercise, and mental health, all of which help uncover patterns in the data.
However, it's vital to recognize the 22% margin of error that remains. Individual circumstances may not be fully captured by the model, leading to variability in predictions.
Here are three key points to reflect on regarding prediction accuracy:
- The model's predictions are based on historical data from 2008 to 2020, focusing on life events recorded between 2008 and 2016.
- Life2vec employs a structured vector space to code life events, allowing for nuanced analysis.
- The model's reliance on diverse life factors contributes considerably to its overall reliability.
Future of Predictive Technologies
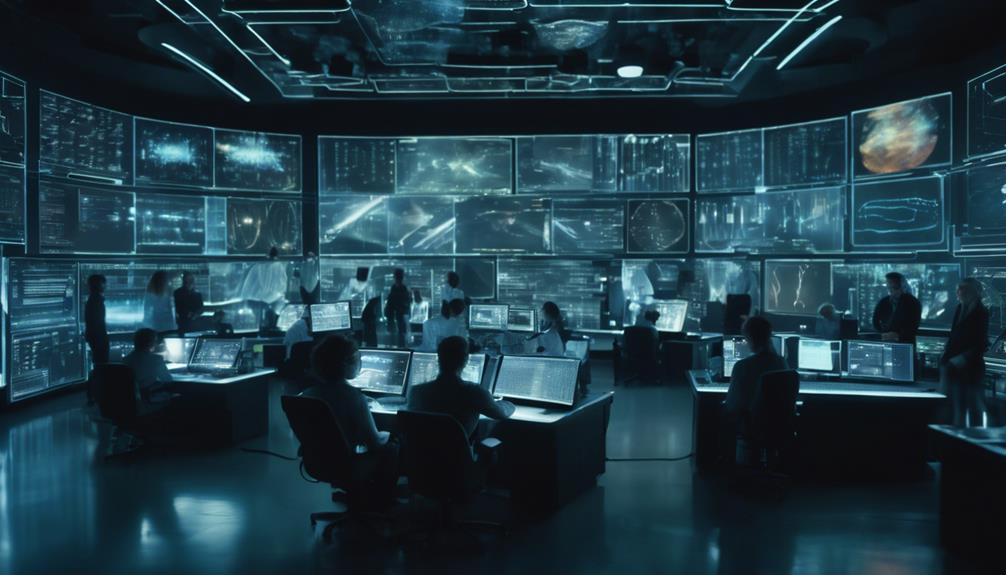
The future of predictive technologies promises to revolutionize how you understand health and personal decisions by integrating diverse data sources for more accurate insights.
With advancements in artificial intelligence, tools like the Life2vec AI model are setting a new standard. It achieved a 78% accuracy rate in predicting life expectancy and financial status at death, analyzing data from over 6 million individuals.
As these technologies evolve, you can expect the integration of text and images, enhancing the applicability of predictions in healthcare and personal insights. Ongoing research is delving into AI applications that personalize medicine through predictive analytics. This shift focuses not only on mortality predictions but also on broader life-events, helping you make informed decisions.
However, as these predictive technologies gain traction, ethical guidelines will be essential. Concerns regarding privacy, data misuse, and the psychological impacts on individuals aware of their predictions must be addressed.
The growing interest in AI tools signals a transformative shift in decision-making and resource allocation, ultimately enriching your understanding of both personal and health-related choices.
Frequently Asked Questions
Is There AI That Can Predict the Future?
Yes, there are AI models designed to predict future outcomes based on historical data. They analyze patterns in various factors, but their accuracy isn't guaranteed, and ethical concerns often arise regarding privacy and potential misuse.
What Is the Name of the AI Predict Death Calculator?
Picture a crystal ball disclosing secrets of mortality; the AI death calculator you're curious about is called Life2vec. It predicts life expectancy and financial status, using data from millions to expose your future.
Is AI Prediction Accurate?
AI predictions can be accurate, but they're not infallible. You need to contemplate the data quality and context, as outcomes can vary due to numerous factors, leading to possible inaccuracies in individual predictions.
What AI Technique Is Used to Predict?
The AI technique used for predictions is called the Life2vec model. It employs transformer models to analyze large datasets, mimicking human brain functions to identify patterns and improve accuracy in forecasting life outcomes.
Can the AI that can predict the future also time travel?
While ai time travel creation might seem like a concept straight out of science fiction, the ability of AI to predict the future does not necessarily mean it can also time travel. Predicting future outcomes is based on analyzing data and patterns, whereas time travel involves manipulating the fabric of spacetime, a complex and theoretical concept.
Conclusion
As you stand on the precipice of a future shaped by predictive AI, it's hard not to feel a thrill of anticipation.
This technology, like a crystal ball, offers glimpses into what lies ahead, but it also raises questions that echo in the corridors of ethics and trust.
While its accuracy dazzles, it's essential to tread carefully, ensuring we harness this power wisely.
Embrace the potential, but remain vigilant; the future's canvas is yours to paint.