Generative AI is transforming language in ways that might surprise you. It creates new linguistic forms, leading to concerns among linguists about innovation versus imitation. While it can blend languages and foster diversity, it risks marginalizing low-resource languages. The AI's reliance on dominant languages can distort cultural nuances and threaten unique expressions. These developments challenge traditional notions of grammar and authenticity, making the understanding of language evolution even more complex. If you're curious about how these changes impact our communication landscape and the future of languages, you'll find some intriguing insights ahead.
Key Takeaways
- Generative AI models can blend syntax and vocabulary from multiple languages, creating novel linguistic outputs that may resemble a new language.
- Linguists are concerned whether AI-generated language represents genuine innovation or mere mimicry of existing language structures.
- The rise of hybrid languages from AI may lead to confusion and miscommunication, particularly among low-resource language speakers.
- Cultural biases in AI training predominantly favor English, risking the marginalization of diverse languages and their unique expressions.
Overview of Generative AI
Generative AI encompasses cutting-edge technologies like ChatGPT and DALL-E that leverage machine learning to create content, making it increasingly difficult to distinguish between human and machine-generated outputs.
At its core, generative AI relies on large language models (LLMs), which analyze vast datasets to learn language patterns. These models generate coherent text based on context and input prompts, demonstrating the potential of AI to mimic human creativity.
The transformer architecture is essential for LLMs, incorporating an attention mechanism that helps maintain context and relevance across extensive texts. This capability allows generative AI to produce plausible responses, yet it often raises concerns about the factual accuracy of the information generated. The focus on generating plausible rather than accurate content challenges our understanding of reliability in AI outputs.
As generative AI continues to evolve rapidly, it's essential for you to grasp its inner workings. Access to educational resources will enhance your comprehension of how these technologies operate and their implications for communication.
Linguistic Challenges and Innovations
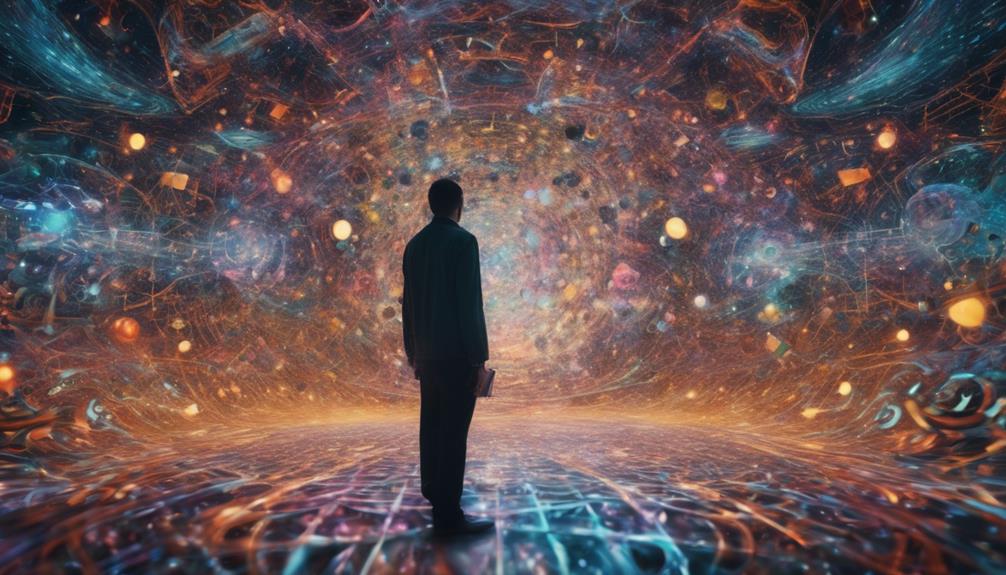
As you explore the landscape of generative AI, you'll encounter both linguistic challenges and innovations that shape language creation dynamics.
It's essential to recognize how AI can either support or hinder the development of lesser-known languages.
Language Creation Dynamics
The rise of AI language models presents both exciting innovations and significant challenges in the domain of language creation. As you explore this landscape, you'll notice that generative AI, particularly large language models (LLMs), can produce novel linguistic outputs. However, these outputs often mimic human syntax without true understanding or creativity. This raises questions about whether we're witnessing genuine linguistic innovation or just sophisticated mimicry.
One challenge you might encounter is the creation of hybrid languages or unique constructs, as AI combines elements from diverse sources, disregarding traditional grammatical rules. This can lead to confusion and miscommunication, especially with low-resource languages, where nuances and cultural contexts often get lost in translation.
While AI language models can contribute to linguistic diversity, they can also threaten the preservation of languages that are already at risk. Ongoing research is vital to understanding how these technologies influence language evolution. You'll find that addressing these linguistic challenges is essential for ensuring that the benefits of AI language generation don't come at the cost of losing the rich tapestry of human languages.
AI Linguistic Innovations
AI language models are pushing the boundaries of linguistic innovation by blending elements from various languages and creating new forms of expression. These models showcase the potential for generating humanlike language, which often leads to fascinating linguistic innovations. However, challenges persist, especially for underrepresented languages.
Here's a quick overview of some key aspects of AI linguistic innovations:
Aspect | Description | Impact |
---|---|---|
Language Blending | Merging syntax and vocabulary from multiple tongues | Expands communication possibilities |
Low-Resource Languages | Focusing on languages with limited training data | Helps combat language extinction |
Ethical Concerns | Issues of authenticity and ownership of AI content | Calls for clearer guidelines in linguistics |
Transformational Grammar | Shift from traditional to generative grammar | Enhances adaptability in language modeling |
As you explore these innovations, remember that while AI models can produce remarkable outputs, they may still lack genuine understanding. The evolution of AI in linguistics not only enriches communication but also highlights the need for ethical considerations as we navigate this complex landscape.
The Role of Traditional Grammar
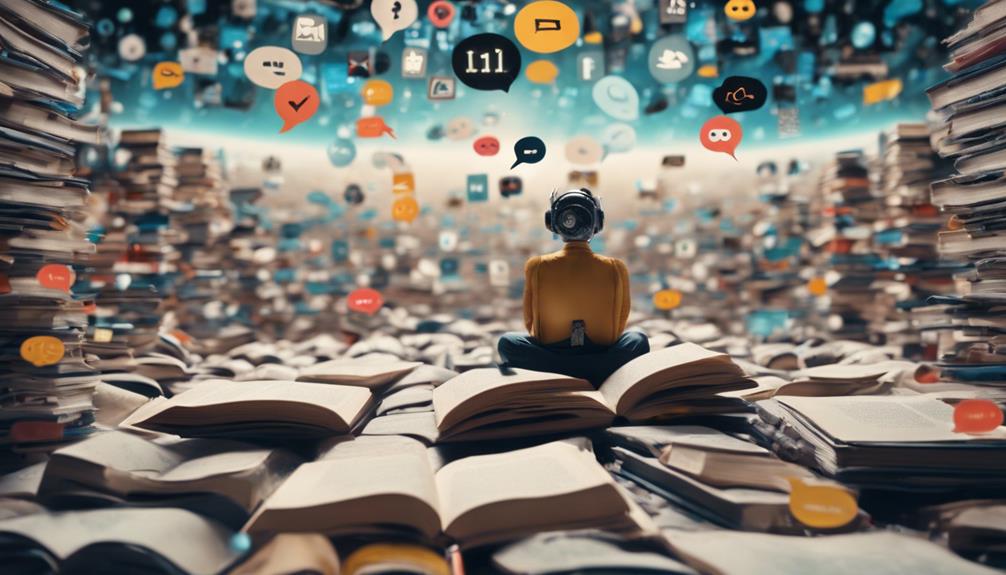
Understanding traditional grammar provides a significant foundation for exploring how language structures influence generative AI's ability to analyze and create sentences. Traditional Grammar serves as a prescriptive framework, emphasizing rules based on opinions rather than the natural evolution of language. In AI research, recognizing these rules is essential because they inform how generative models interpret and produce language.
Contrasting with Traditional Grammar is Transformational Generative Grammar (TGG), developed by Noam Chomsky. TGG focuses on the generative rules that create grammatical sentences by analyzing sentence elements and their context. This emphasis on inherent rules revolutionized our understanding of language, moving beyond simple descriptions.
The principles of Traditional Grammar have historically shaped educational approaches in language arts, sparking debates on effective teaching methods. By understanding these foundational concepts, you can better appreciate how generative AI models mirror linguistic rules.
These models learn from vast datasets, identifying patterns that reflect traditional structures while also innovating new combinations. As AI continues to evolve, the interplay between traditional grammar and generative processes will be important in shaping future language understanding and production.
Cultural Biases in AI Language
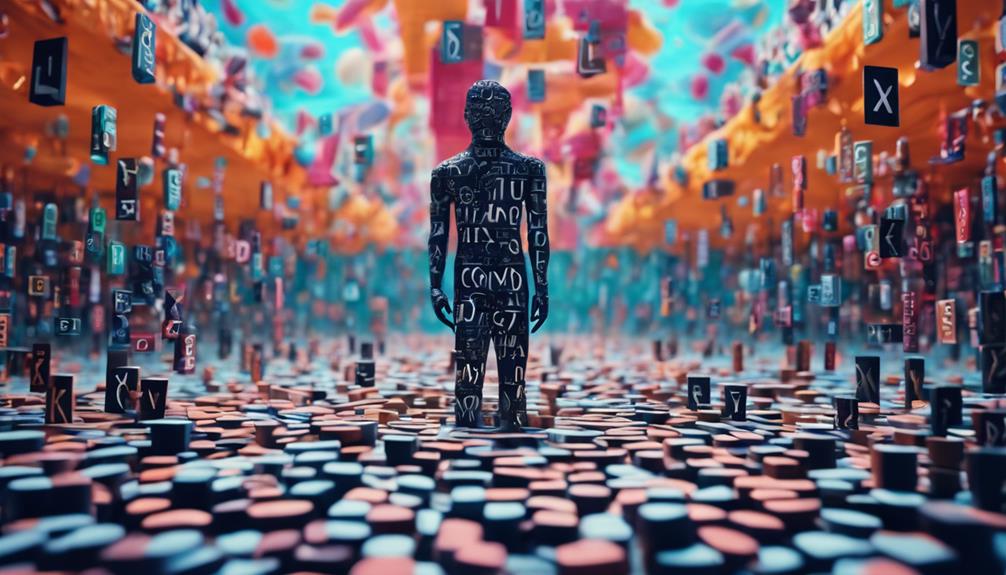
When you look at how AI handles language, it's clear that many languages, especially Indigenous ones, get sidelined.
With most AI models trained primarily on English, you're seeing a significant cultural bias that overlooks the richness of diverse languages.
This not only misrepresents these languages but also marginalizes communities that rely on them for communication.
Language Representation Issues
Cultural biases in language representation often lead to the erasure of many languages and their speakers, sidelining the rich diversity of human communication. You mightn't realize how these biases manifest in AI, impacting communities globally.
Here are a few key points to contemplate:
- Generative AI frequently misclassifies languages like Fon as fictional, showcasing cultural invisibility.
- Over 80% of the world doesn't speak English, yet AI training relies heavily on English text, marginalizing low-resource languages.
These language representation issues highlight a critical gap in AI development. The lack of digital resources for low-resource languages means that many are overlooked.
When AI prioritizes Western knowledge, it neglects local contexts, risking misrepresentation and misinformation. This can distort the understanding of diverse cultures and their languages, causing further marginalization.
The limited support for languages like Fon and others reflects a broader problem in recognizing the richness of human expression.
To foster inclusivity, we need to advocate for the incorporation of all languages into AI training, ensuring every voice is heard and valued.
Marginalization of Indigenous Languages
The marginalization of Indigenous languages in AI underscores a pressing issue, as countless speakers find their voices overlooked in a technology landscape dominated by English. Despite over 80% of the global population not speaking English, generative AI is primarily trained on English text. This cultural bias not only reinforces the marginalization of Indigenous languages but also impacts their speakers who struggle to access resources and tools.
When you consider that only 10 languages account for nearly 90% of website content, it's clear that many Indigenous and less widely spoken languages are at risk of extinction. Misclassifying languages like Fon as fictional further highlights the cultural invisibility faced by these communities. The lack of quality training data for low-resource languages leads to poor translations and miscommunication, which can alienate speakers and diminish their language's relevance.
To combat this marginalization, we must advocate for inclusive AI training data that represents diverse languages and cultures. By doing so, you can help guarantee that Indigenous languages not only survive but thrive in the digital age.
It's crucial for technology to reflect the richness of human language and culture.
Effects on Language Diversity
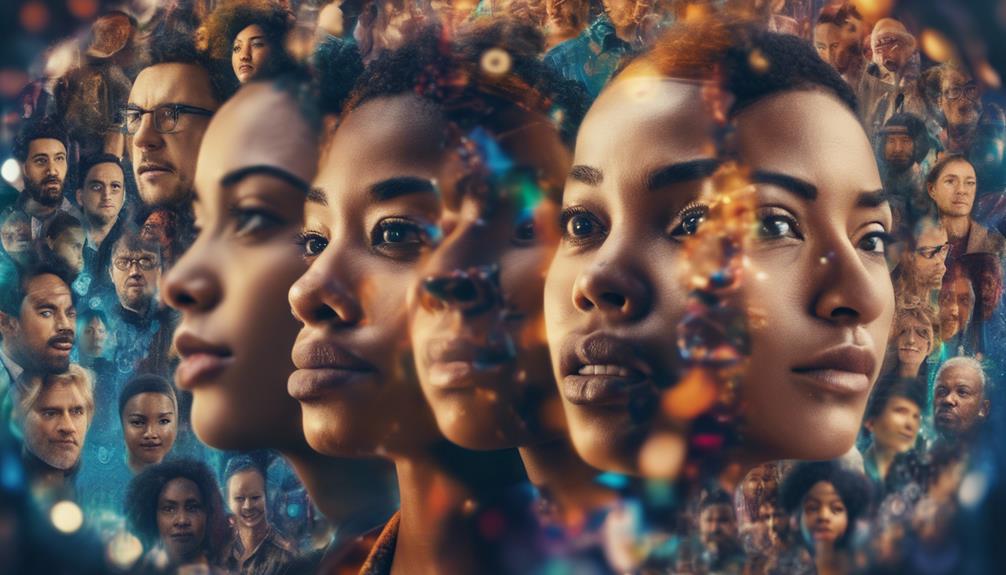
Generative AI is narrowing language diversity by primarily focusing on dominant languages, sidelining countless Indigenous and low-resource languages in the process. This trend reinforces cultural biases, leading to a significant imbalance in representation and understanding.
Consider these impacts:
- Limited Training: AI models excel in just 8-10 high-performance languages, leaving approximately 7,000 languages at risk of being ignored.
- Cultural Loss: Poor translations in low-resource languages often result in misinformation, eroding essential cultural nuances.
As a result, language diversity suffers. The scarcity of quality training data for low-resource languages hinders accurate comprehension, creating barriers to effective communication.
The focus on dominant languages not only marginalizes speakers of less common languages but also diminishes the rich tapestry of human expression. If this trend continues, the cultural and linguistic wealth that comes from diverse languages may be lost forever, leaving a homogenized digital landscape devoid of the unique voices that enrich our world.
Language Preservation Initiatives
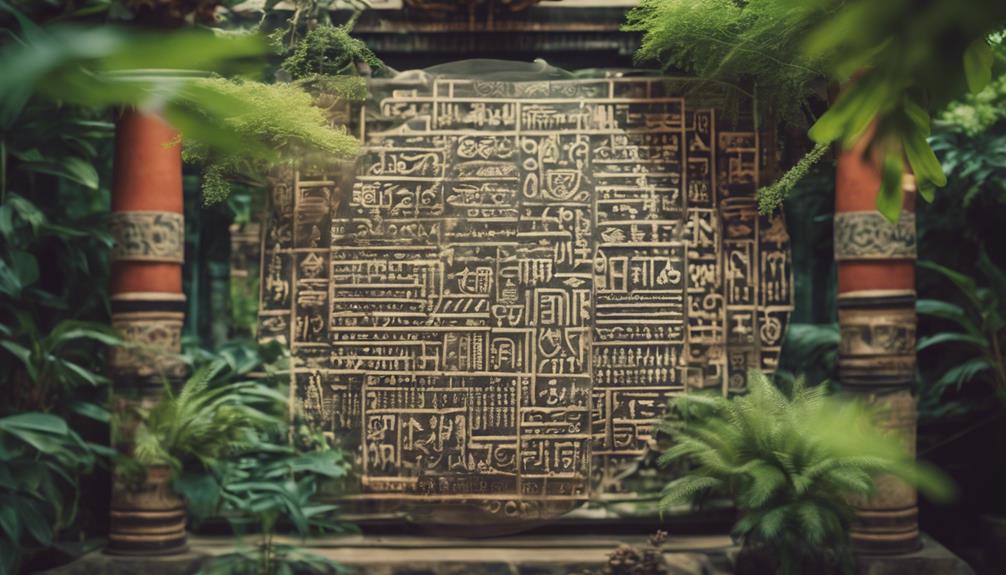
Language preservation initiatives are gaining momentum as communities recognize the urgent need to protect and revitalize their linguistic heritage.
You're likely aware that AI technologies play an important role in these efforts, especially in underrepresented linguistic communities. For instance, the Masakhane initiative focuses on developing AI tools specifically for African languages, enabling better communication and understanding among speakers.
Community involvement is essential in language preservation, as it helps identify the specific needs and features for effective language model development. Engaging native speakers in collaborative efforts to curate training data guarantees that the resources created are accurate and relevant, though it often demands substantial time and effort.
Despite platforms like Fon Wikipedia, a significant disparity remains in the number of articles compared to English, highlighting the need for more robust language resources.
By leveraging AI technologies alongside community involvement, you can contribute to creating better tools that support linguistic diversity. Together, these initiatives can foster a deeper appreciation for cultural heritage and encourage younger generations to engage with their languages.
It's an exciting time for language preservation, and your participation can make a real difference.
Future of AI and Linguistics
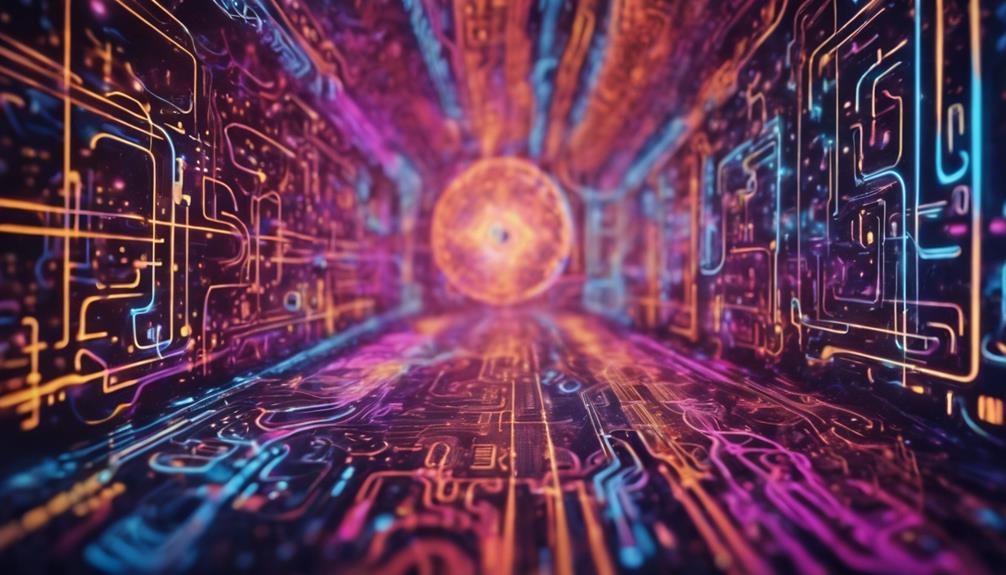
Exploring the future of AI in linguistics reveals exciting possibilities for more nuanced understanding and interaction with languages. You'll find that the intersection of AI and linguistics is paving the way for innovative methodologies and applications. Some key areas to watch include:
- Advanced language models: With tools like Cohere performing well in 101 languages, the potential for broader language support is huge.
- Generative AI advancements: Techniques such as Transformers and Attention Mechanisms are transforming how we analyze linguistic patterns, enhancing automated content creation.
As AI continues to evolve, it's crucial to reflect on the ethical implications of AI-generated language. Ongoing debates highlight the need for interdisciplinary collaboration between linguists and technologists.
This partnership can help navigate the complex landscape of language and technology, ensuring that future AI language models respect linguistic diversity and cultural significance. By embracing these advancements, you can actively participate in shaping a future where AI enriches our understanding of linguistics.
Frequently Asked Questions
What Are the Negative Effects of Generative Ai?
Generative AI can perpetuate cultural biases, misclassify languages, and create content saturation. It often neglects low-resource languages, leading to misinformation and loss of cultural nuances, ultimately diminishing the quality and diversity of language resources available.
Can AI Generate New Languages?
Did you know that over 90% of languages remain undocumented? While AI can generate new linguistic patterns, it often lacks the depth and cultural nuances that human language embodies, limiting its ability to create truly original languages.
Will AI Take Over Linguistics?
You might wonder if AI will take over linguistics. While AI can mimic language, it lacks true creativity and understanding. Linguistic expertise remains essential, ensuring that human language's depth and diversity continues to thrive.
Can We Use Generative AI for Language Translation?
Yes, you can use generative AI for language translation, but be cautious. While it enhances translations for certain languages, many low-resource languages still lack support, leading to potential inaccuracies and cultural biases in the results.
Conclusion
As you ponder the future of language shaped by generative AI, think of a garden.
Just like a gardener cultivates diverse plants, we must nurture linguistic variety amidst AI's rapid growth. Each unique flower represents a language or dialect, each with its own beauty and history.
If we ignore these blooms, we risk a monoculture where richness fades.
By embracing AI's innovations while protecting our linguistic heritage, we can guarantee a vibrant tapestry of communication for generations to come.